Recently, CNNIC (China Internet Network Information Center) released a statistical report on the status of the latest Chinese network development, indicating that AI technology is driving the upgrading of the education industry. In this wave, a large number of educational companies are doing their best to join the camp of AI+ education in the hope of becoming the first “king†to eat crabs. This article was compiled by Lei Fengnet and compiled by Pearson Group on the AI+ education research report "Intelligence Unleashed: an argument for AI in education" by Rose Luckin, Wayne Holmes, UCL (University College London), and Pearson Group. Mark Griffiths, Laurie B. Forcier.
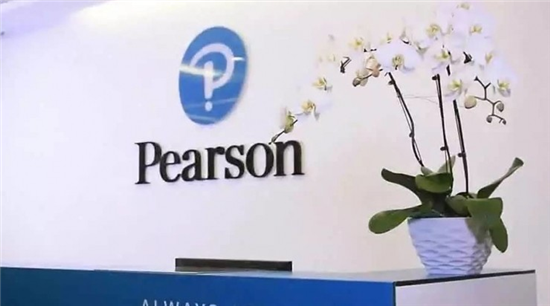
As we all know, many applications of AI+ education have been used by institutions such as schools, middle schools and primary schools. Many AI+ education and educational data mining (EDM) technologies are also used to track student behavior.
For example, by collecting student attendance and task submission data in class, students can learn whether they are in an unmotivated state of learning. There are other AI researchers who are exploring more creative user interfaces such as NLP (natural language processing), speech and gestures, eye tracking, and other physical sensors.
However, in this article, we will focus on three types of AI+ educational software applications that can be used directly to support learning:
? Provide learners with personalized guidance;
? Provide intelligent support for collaborative learning;
Virtual reality assisted learning.
Adaptive System 丨 AI+ Education can provide intelligent, personalized guidance for everyone
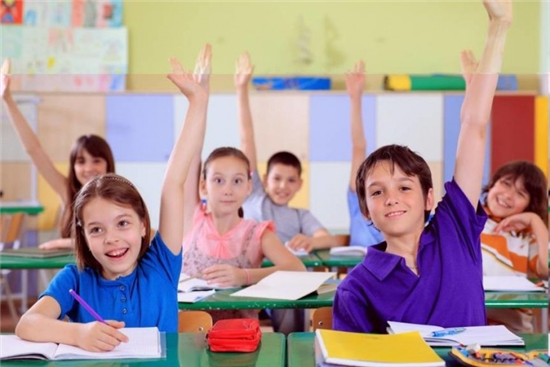
In physical education, one-on-one coaching is often considered the most effective way. However, this method does not fully apply to all students. There are two reasons: As a result, there are not so many teachers. Secondly, students cannot afford such high tuition.
So this raises a problem for everyone: how can we provide the positive impact of one-on-one coaching to all students in all subjects.
This is where ITS (Intelligent Tutoring System) accesses. ITS uses AI technology to simulate one-on-one instruction, provide learning tasks that best suit the cognitive needs of learners, and provide targeted and timely feedback. This process does not require the attendance of teachers.
Some ITS systems can also help trainees to manage their learning status and develop self-discipline. Some people can use teaching strategies to manage learning so that they can get appropriate challenges and support in their studies.
In the 1970s, the first AI systems provided personalized and adaptive guidance. Take BUGGY as an example, this is a groundbreaking system designed to guide addition and subtraction, using a model that the learner may confuse in program operations. This "bad library" is an effective leading model in the system. It is used to diagnose every mistake made by the students so as to provide appropriate guidance. Initially, it was limited by identifiable bugs that were already included in the original code. Over time, additional misunderstandings were discovered and added to this "error store."
In addition to these models, many recent ITS systems also use machine learning techniques, self-training algorithms based on large data sets, and neural networks that provide these learners with what they are learning and make appropriate decisions. However, with this approach, it is still difficult to make these decisions rational and clear.
By contrast, adaptive systems based on modern models are much more flexible. They allow every decision made by the system to be properly understood by humans and thus more suitable for classroom teaching. Over the past decade, with the increasing complexity of learners and educators, leading models have also been introduced into many adaptive systems to support personalized learning recommendations.
For example, the iTalk2Learn system is designed to help young students learn the “score†part of mathematics and use a learner model that includes the learner’s level of mathematical knowledge, cognitive needs, emotional state, and feedback they receive. Responses to feedback and other information.
Based on adaptive learning can include a series of tools for AI+ education:
Learner's cognitive and emotional state model.
• Use conversations to engage students in learning experiences that involve inquiry and discussion, questions, and answers.
Includes an open learner model to promote learner reflection and self-awareness.
• Use a metacognitive framework (eg, by providing dynamic help or using a narrative framework) to enhance learner motivation and engagement.
• Use social simulation models – For example, by understanding social norms and cultures, students of language learning can better communicate with the speakers of the target language.
Collaborative Learning 丨 AI+ Education Provides Smart Support for Collaborative Learning
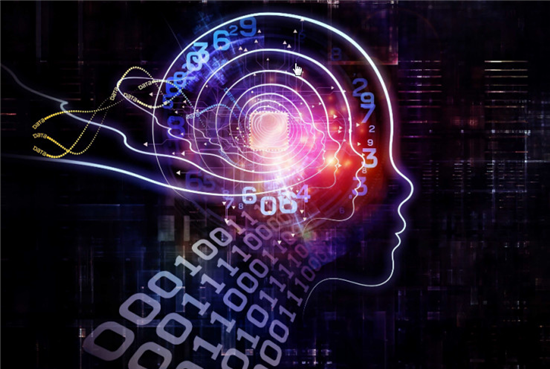
Decades of research have shown that for collaborative learning, both students who join the project together, or those who participate in online courses, have better learning outcomes than those who learn independently. . Collaborative learning is effective because it encourages participants to express and strengthen their ideas, eliminate disagreements through constructive dialogue, and present a new understanding of shared knowledge. In addition, collaborative learning can enhance motivation - if students focus on the group they are involved in, they will be more actively involved in this task and achieve better learning outcomes.
However, studies have also shown that collaborative learning among learners does not occur spontaneously. For example, team members may not have the social interaction skills needed for collaboration. This is especially difficult in the context of the offline communication between collaborators collaborating online. This is where AI+ education can come in. According to the results of our investigation, we mainly focused on the following four aspects: the formation of an adaptive group; expert facilitation; intelligent virtual assistants; intelligent auditing.
The formation of adaptive teams
The system typically uses AI techniques and knowledge to manage independent participants, most commonly learner models, to form the group that best fits the specific collaborative task. The purpose may be to allow students in this group to have similarities in cognitive level and interest, or participants can bring each other different but complementary knowledge and skills.
Expert simplification
These effective cooperation models, collectively referred to as "cooperative models," are generally used to provide interactive knowledge to collaborative students. These patterns are provided by the system authors and derived from previous collaborations. For example, AI technologies such as machine learning and Markov models have been used to identify collaborative strategies that effectively solve problems. These can be used to train the system to help students better understand the knowledge and concepts shared with each other, or to provide targeted support for the right form at the right time.
Smart Virtual Assistant
This method involves the introduction of intelligent virtual assistants into the collaboration process. These AI assistants may mediate students' online interactions or simply facilitate conversations by:
Expert participants (a coach or tutor)
? Virtual partner (similar to a virtual classmate who is at the same cognitive level as the student, but this small partner can introduce novel ideas)
Or students themselves - for example, virtual partners may have problems with misunderstandings, thereby providing students with alternative perspectives to stimulate more creative arguments and reflective output.
Smart audit
Because there are a large number of students working in multiple collaborative groups, it is impossible for one person to understand the large amount of data that the participants generate during the discussion. At this time, smart audit methods are needed. It analyzes and summarizes this discussion through AI techniques, such as machine learning and shallow text processing, so that human teachers can guide students in effective collaboration. For example, the system can provide alerts to human teachers to inform them of major events that may require their intervention or support, such as the student leaving the subject or making repeated mistakes while doing the problem.
We had some simple goals: design a caulking gun that was efficient, durable, functional, and lightweight. Finally, after many prototypes, trials, and false starts, the Siligun series of caulking guns was brought to life!
The right caulking gun for you depends on the type of caulk you will be applying, as well as the overall scope of your project.
Siligun Sealant Gun,Sealant Gun,Manual Sealant Gun,Sealant Gun 600Ml
jiangsu concentric internatinal ltd , https://www.jsconcentriccorps.com